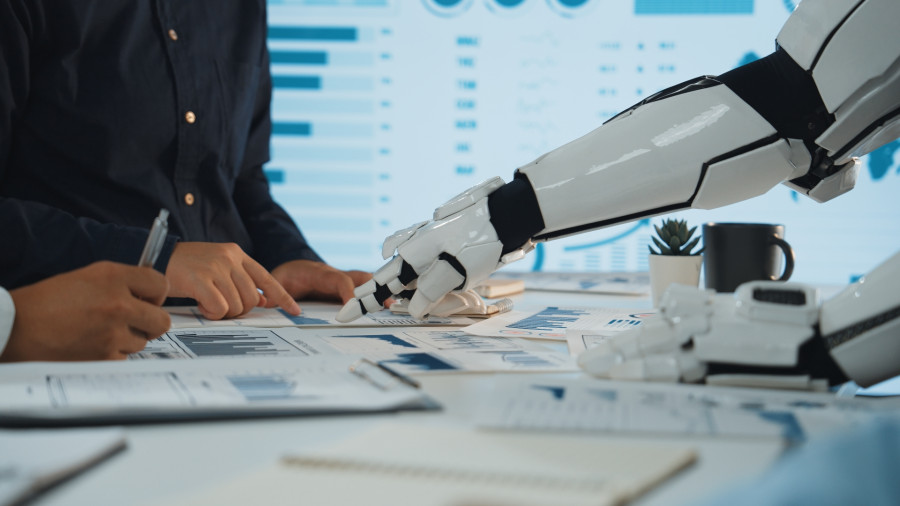
When it comes to identifying winning investments, data-driven methods may outperform both economic models and professional analysts, according to HKUST’s Yang Ha (Tony) Cho and colleagues. They sought to “push the frontier of earnings prediction” by “applying machine learning methods to a large set of detailed financial data” for the first time.
Earnings are notoriously difficult to predict using firms’ current performance, even for experienced investors. “Earnings forecasts based on firm characteristics,” the researchers note, “are not substantially more accurate than forecasts obtained from the random-walk model.” Traditional mathematical models, using regression techniques on limited financial data, struggle with the vagaries of real markets.
Advances in computing and data availability are unlocking new approaches to thorny prediction problems. The researchers exploited this emerging toolset by applying two of the most successful machine learning (ML) methods to predict earnings changes for a range of firms. Although “future earnings are difficult to forecast as they are related to numerous aspects of a company in a complex manner,” ML has the potential to step in where academic theories fail to deliver.
Both the tested ML methods—random forest and stochastic gradient boosting—were highly accurate in forecasting the direction of earnings changes. Indeed, ML considerably outperformed regression—as well as financial analysts—in predicting whether firms’ earnings would rise or fall over time. An investor guided by the ML results would have obtained significantly better returns than one relying on regression models or experts with access to specialist knowledge.
Cho and colleagues chalk up the success of ML to two factors. First, high-power computers can crunch huge sets of financial numbers. Moreover, unlike classical models, they can analyze complex nonlinear relationships between various types of data. “Our detailed financial data offer more nuanced information,” they conclude, “and unleash the power of machine learning.”